Sophie Lemmens,a,b Lies De Groef,c Wouter Charle,d Murali Jayapala,d Jan Theunis,e Lieve Moons,c Patrick De Boeverf and Ingeborg Stalmansa,b
aKU Leuven, Biomedical Sciences Group, Department of Neurosciences, Research Group Ophthalmology, Herestraat 49, Leuven, 3000, Belgium
bUniversity Hospitals UZ Leuven, Department of Ophthalmology, Herestraat 49, Leuven, 3000, Belgium
cNeural Circuit Development and Regeneration Research Group, KU Leuven, Belgium
dHyperspectral Imaging Technology Research Group, imec, Belgium
eHealth Unit, VITO, Belgium
fCentre of Excellence Microbial Systems Technology, University of Antwerp, Belgium, and Centre for Environmental Sciences, Hasselt University, Belgium
DOI: https://doi.org/10.1255/sew.2021.a26
© 2021 The Author
Published under a Creative Commons BY-NC-ND licence
In a recent multidisciplinary study involving 39 patients, the potential of retinal imaging techniques for the diagnosis of Alzheimer’s disease was investigated. An easy-to-use hyperspectral snapshot camera—16 spectral bands between 460 nm and 620 nm with 10 nm bandwidth—was used to quantify amyloid accumulation, while optical coherence tomography allowed the thickness of the retinal nerve fibre layer to be assessed. Dedicated image preprocessing and machine learning were instrumental in discriminating between Alzheimer patients and healthy subjects. The best results were obtained when the hyperspectral and OCT data were combined.
Alzheimer’s Disease and its biomarkers
Today, Alzheimer’s disease (AD) is diagnosed based on (the combination of) three biomarkers: amyloid-beta (Aβ) and tau protein accumulation and neurodegeneration parameters.1 Data are assembled by performing positron emission tomography scans or analysing cerebrospinal fluid; both are costly and/or invasive procedures. An affordable and non-invasive diagnostic test for these biomarkers is desirable.
The eye is closely related to the brain and spinal cord. Therefore, it provides a unique window into the central nervous system. One research route towards non-invasive AD diagnosis focuses on retinal examinations. In transgenic mouse models of AD, Aβ accumulation and plaques were observed in the retina before being present in the brain.2 There is accumulating evidence in AD patients for the presence of AD disease hallmarks in the retina.3 Different imaging techniques are being studied to detect retinal changes related to Aβ presence, in vivo. One of these techniques is hyperspectral retinal imaging, with wavelengths between 460 nm and 570 nm being the most interesting to use.4,5 Post-mortem studies in both animal and human retinas, and in vivo studies in rodents, have shown that hyperspectral retinal imaging can detect spectral changes that could be caused by the presence of retinal Aβ aggregates.4,6 Hyperspectral retinal imaging, however, does not directly visualise retinal Aβ deposition, but records a spectral shift at wavelengths between 460 nm and 570 nm that could be explained by the presence of retinal protein deposits in certain stages of aggregation, given the relationship between particle size and different types of light scattering.
An important neurodegeneration biomarker, in the eye, is the thinning of the retinal nerve fibre layer. This can be studied by optical coherence tomography (OCT). This non-invasive and high-resolution tool produces cross-sections of the retina.7
From June to September 2019, a study with 39 patients was performed at the Ophthalmology Department of the University Hospital UZ Leuven in Belgium (Figures 1 and 2). Seventeen patients were recruited from the Memory Clinic of UZ Leuven, 10 with clinically-probable AD and 7 with biomarker-proven AD. Also, 22 controls were included in the study.
Figure 1. Patient being examined using retinal hyperspectral imaging and optical coherence tomography.
Figure 2. Study set-up.
Hyperspectral imaging of the retina for amyloid quantification
The hyperspectral retinal imaging was performed with a snapshot camera from XIMEA (SNm4x4 VIS), connected to a C-mount to a TL-230T relay lens from Topcon on a Topcon TRC-50DX fundus camera.
At the heart of the XIMEA camera is a hyperspectral sensor from imec. The sensor has a standard CMOS 1088 × 2048 pixel image sensor as a base with mosaic patterned hyperspectral filters post-processed on top of it. This dedicated design allows spatial and spectral data to be acquired in one capture without the need for scanning.8 4 × 4 imaging pixels are combined into hyperspectral pixels with 16 spectral bands of 10 nm bandwidth between 460 nm and 620 nm.
An exposure time of 0.2 ms, a 50 ° field of view and no background illumination were used to acquire the images. Patients were subjected to one short flash of low to moderate intensity while focusing on an external fixation light.
Relative reflectance was computed for each hyperspectral image. Further, blood vessels were removed from the hyperspectral images by applying a difference of Gaussians filter to the entire greyscale image. Finally, four regions of interest (ROIs) were defined for standardisation purposes, based on the centre of the optic disc (Figure 3). This strategy is a compromise between considering the entire retina, with the risk to dilute a possibly weak Aβ signal, and considering a large number of regions, with the risk of detecting random effects.
Figure 3. Left: Four regions of interest were defined: superior 1 (S1), superior 2 (S2), inferior 1 (I1) and inferior 2 (I2). The green parts in the image are the ones used for the analysis, with the retinal blood vessels substracted from the image. Right: Mean spectra in the four regions of interest. Shaded areas indicate the mean ± the standard error of the mean.
Optical coherence tomography to visualise the retinal nerve fibre layer thickness
An RT-vue XR Avanti from Optovue was used to perform the OCT analysis and calculate the retinal nerve fibre thickness. This was done both over 360 ° and per quadrant.
Machine learning, using HSI and OCT data
Classification models were developed based on linear discriminant analysis (LDA). Models were trained using scikit-learn library (version 0.21.3) in a Python programming language environment.
For each region of interest, two input configurations for the classifier were evaluated: one that consisted of normalised hyperspectral data and one that combined normalised hyperspectral data and optical coherence tomography features.
Results
The resulting models could discriminate between AD subjects and controls with an accuracy of approximately 75 % in a nested leave-one-out cross-validation. Out of the four configurations depicted in the image below, the best one (I2 + RNFL), was trained and evaluated, resulting in an area under the curve of 0.74 (95 % CI [0.60, 0.89]) (Figure 4). The hyperspectral information present in the images was the main driver for this classification result. The classification accuracy improved by including OCT data.9
Figure 4. Left: average receiving operating characteristic (ROC) curves over all inner loop cross-validation runs for all configurations. Right: average ROC curve over all outer loop cross-validation runs for the I2 + RNFL configuration, which showed the best performance in the inner loop.
Conclusion
The bi-modal imaging approach using hyperspectral and OCT data resulted in a successful proof-of-concept to detect amyloid-beta changes in the retina of Alzheimer patients. The data were used to train a model which could discriminate AD patients from controls with 80 % accuracy. The accuracy of the model improved when adding the OCT data, a measure of retinal nerve fibre layer thickness.
This study shows the potential of using retinal imaging techniques, based on hyperspectral imaging, for a non-invasive, faster and low-cost diagnostic test for AD than is available today. The snapshot camera used in this study is especially interesting because the spatial and spectral information can be obtained in one take, enabling real-time data acquisition. This is essential to deal with the eye movements, and a key enabler for this application.
Future studies are needed with more patients to confirm the results of this pilot study.
Acknowledgements
This research was carried out in the framework of the ADMIRE project (Alzheimer’s Disease Detection Using Multimodal Imaging of the Retina) and funded through the Mission Lucidity initiative (https://www.missionlucidity.com).
The PhD of Sophie Lemmens was jointly funded by VITO and KU Leuven.
Part of this research work has been co-funded in the context of the HERALD project that was granted by the ATTRACT consortium, which received funding from the European Union’s Horizon 2020 Research and Innovation Programme (2014–2020).
Further, research is funded through a JNPD grant with the University of Melbourne and Umea University, and through a joint PhD programme from the KU Leuven and the University of Melbourne.
References
- C.R. Jack, D.A. Bennett, K. Blennow, M.C. Carrillo, H.H. Feldman, G.B. Frisoni, H. Hampel, W.J. Jagust, K.A. Johnson, D.S. Knopman, R.C. Petersen, P. Scheltens, R.A. Sperling and B. Dubois “A/T/N: an unbiased descriptive classification scheme for Alzheimer disease biomarkers”, Neurology 87(5), 539–547 (2016). https://doi.org/10.1212/WNL.0000000000002923
- M. Vandenabeele, L. Veys, S. Lemmens, X. Hadoux, G. Gelders, L. Masin, L. Serneels, J. Theunis, T. Saito, T.C. Saido, M. Jayapala, P. De Boever, B. De Strooper, I. Stalmans, P. van Wijngaarden, L. Moons and L. De Groef, “The AppNL-G-F mouse retina is a site for preclinical Alzheimer’s disease diagnosis and research”, Acta Neuropath. Commun. 9, 6 (2021). https://doi.org/10.1186/s40478-020-01102-5
- N. Mirzaei, H. Shi, M. Oviatt, J. Doustar, A. Rentsendorj, D.-T. Fuchs, J. Sheyn, K.L. Black, Y. Koronyo and M. Koronyo-Hamaoui, “Alzheimer’s retinopathy: seeing disease in the eyes”, Front. Neurosci. 14, 921 (2020). https://doi.org/10.3389/fnins.2020.00921
- S.S. More, J.M. Beach and R. Vince, “Early detection of amyloidopathy in Alzheimer’s mice by hyperspectral endoscopy”, Investig. Ophthalmol. Vis. Sci. 57, 3231–3238 (2016). https://doi.org/10.1167/iovs.15-17406.
- S. Lemmens, J. Van Eijgen, K. Van Keer, J. Jacob, S. Moylett, L. De Groef, T. Vancraenendonck, P. De Boever and I. Stalmans, “Hyperspectral imaging and the retina: worth the wave?”, Trans. Vis. Sci. Tech. 9, 9 (2020). https://doi.org/10.1167/tvst.9.9.9
- S. More and R. Vince, “Hyperspectral imaging signatures detect amyloidopathy in Alzheimers mouse retina well before onset of cognitive decline”, ACS Chem. Neurosci. 6(2), 306–315 (2015). https://doi.org/10.1021/cn500242z
- J. den Haan, F.D. Verbraak, P.J. Visser and F.H. Bouwman, “Retinal thickness in Alzheimer’s disease: a systematic review and meta-analysis”, Alzheimers Dement. Diagn. Assess. Dis. Monitor. 6, 162–170 (2017). https://doi.org/10.1016/j.dadm.2016.12.014
- A. Lambrechts, P. Gonzalez, B. Geelen, P. Soussan, K. Tack and M. Jayapala, “CMOS-compatible, integrated approach to hyper- and multispectral imaging”, 2014 IEEE International Electron Devices Meeting, pp. 10.5.1–10.5.4 (2014). https://doi.org/10.1109/IEDM.2014.7047025
- S. Lemmens, T. Van Craenendonck, J. Van Eijgen, L. De Groef, R. Bruffaerts, D.A. de Jesus, W. Charle, M. Jayapala, G. Sunaric-Mégevand, A. Standaert, J. Theunis, K. Van Keer, M. Vandenbulcke, L. Moons, R. Vandenberghe, P. De Boever and I. Stalmans, “Combination of snapshot hyperspectral retinal imaging and optical coherence tomography to identify Alzheimer’s disease patients”, Alzheimers Res. Ther. 12, 144 (2020). https://doi.org/10.1186/s13195-020-00715-1
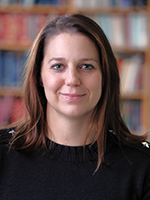
Sophie Lemmens
Sophie Lemmens works as an ophthalmologist in the Research Group Ophthalmology of the University Hospital UZ in Leuven. She holds a Master of Science from KU Leuven and a PhD in Medicine from the same university. Her dissertation was entitled: “The eye as a window to the brain: a quest for retinal biomarkers in neurodegenerative disease”. 0000-0002-6842-1747
sophie.1.lemmens@uzleuven.be
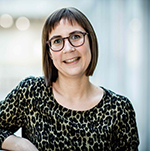
Lies De Groef
Lies De Groef is principal investigator at the Animal Physiology and Neurobiology division of the KU Leuven. She obtained her PhD in Biochemistry & Biotechnology at KU Leuven in 2015. In 2017, she returned to the Neural Circuit Development and Regeneration Research Group, where she established a new research line focusing on the retinal manifestations of Alzheimer’s, Parkinson’s and Wolfram disease. 0000-0002-3329-3474
lies.degroef@kuleuven.be
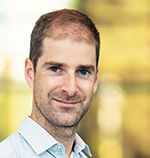
Wouter Charle
Wouter Charle is manager for hyperspectral imaging technology at imec, leading the off-the-shelf and evaluation system activities. He has a background in physics and software engineering. After starting his career in 3D machine vision, he joined imec in 2016 to help growing the hyperspectral imaging business.
wouter.charle@imec.be
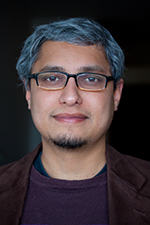
Murali Jayapala
Murali Jayapala is a researcher at imec, focusing on system design aspects for next generation imaging/vision, optics & photonics systems. In 2005, he received his PhD in Applied Sciences (Computer Engineering) from KU Leuven, Belgium. In 1999, he also obtained his M.E. in Systems Science and Automation from Indian Institute of Science (IISc), India. 0000-0001-7917-0149
murali.jayapala@imec.be
Jan Theunis
Jan Theunis is project manager at the Health Unit of VITO, the Flemish Institute of Technological Research. He holds a degree of Master of Science in Bio-Engineering from the Leuven University. In the last 10 years he has been focusing on the integration of new sensor technologies for personal environment and health, and machine learning techniques, in monitoring and prevention applications. 0000-0002-4191-5667
jan.theunis@vito.be
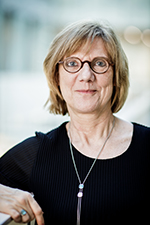
Lieve Moons
Lieve Moons heads the Neural Circuit Development and Regeneration research group within the Biology Department at KU Leuven. She obtained her PhD in Science at KU Leuven in 1990. From 1995 till 2007, she was group leader in the Vesalius Research Center (VRC) at the Flemish Interuniversity Institute for Biotechnology, where she contributed to an extensive research programme in vascular & neural development and in cardiovascular & neurodegenerative disorders. In 2008, she became full professor and head of the Neural Circuit Development and Regeneration (NCDR) research group—established within the Biology Department at KU Leuven—which has a strong interest in defining cellular/molecular mechanisms underlying neurodegeneration, neuroinflammation and regeneration in the injured, diseased or aged central nervous system (CNS) of both teleost fish and rodents. She has profound expertise in morphological, functional and behavioural phenotyping of animal disease models for optic neuropathies and neurodegenerative diseases. 0000-0003-0186-1411
lieve.moons@kuleuven.be
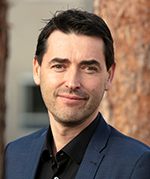
Patrick De Boever
Patrick De Boever is research and innovation manager of the Centre of Excellence Microbial Systems Technology at the University of Antwerp and he has an appointment as lecturer at Hasselt University. He holds a PhD in Applied Biological Sciences from Ghent University and a Master of Science in Bio-Engineering from that same university. From 2007 until 2020, he worked as a project manager at the Health Unit of VITO, the Flemish Institute of Technological Research. He was team leader of the MONA group with a focus on retinal image analysis, with a particular interest in machine learning and artificial intelligence for screening and diagnosis in medical applications. 0000-0002-5197-8215
patrick.deboever@uantwerpen.be
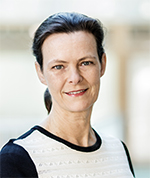
Ingeborg Stalmans
Professor Ingeborg Stalmans heads the Research Group Ophthalmology (RGO) at the KU Leuven as well as the glaucoma unit of the ophthalmology department at UZ Leuven. The current focus of her basic and clinical research work is retinal imaging as a biomarker for systemic diseases. She established the ADMIRE consortium in collaboration with Mission Lucidity (a partnership between KUL and Imec) to accomplish this mission. 0000-0001-7507-4512
ingeborg.stalmans@mac.com