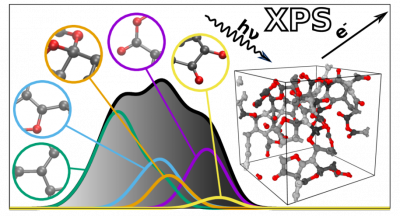
Carbon-based materials hold enormous potential for building a sustainable future, but material scientists need tools to properly analyse their atomic structure, which determines their functional properties. X-ray photoelectron spectroscopy (XPS) is one of the tools used to do this, but XPS results can be challenging to interpret. Now, researchers at Aalto have developed a machine-learning tool to improve XPS analyses, which they have made freely available as the XPS Prediction Server.
XPS spectra reflect the binding energy of the electrons deep in the atoms that make up a material. Because the binding energies depend on the atomic environment, they can be used to infer how the atoms are connected in a particular material or molecule. However, this also makes XPS spectra difficult to interpret, since many factors affect binding energies. The binding energies of different atomic features can also overlap, further complicating the analysis.
To help with this, a team led by Miguel Caro developed a computational method that can predict the binding energy spectrum of a material based on a computer-generated structural model. This simplifies XPS data interpretation by making it possible to match the experimentally observed binding energies against the computational predictions.
The idea itself isn’t new, but the problem has been the computational difficulty of calculating the XPS spectrum of a material accurately. Caro’s team solved this using machine learning. The trick was to train an inexpensive computer algorithm to predict the outcome of a computationally expensive reference method based on an efficient combination of computationally cheap and expensive quantum mechanical data.
The computationally cheaper method, DFT, doesn’t match experimental results very accurately. The more accurate method, GW, takes too long to compute when a molecule has many atoms. “We decided to construct a baseline model that uses abundant DFT data and then refine it with scarce and precious GW data. And it worked!”, says Caro.
The resulting algorithm can predict the spectrum of any disordered material made of carbon, hydrogen and oxygen. “The predicted spectra are remarkably close to those obtained experimentally. This opens the door to better integration between experimental and computational characterisation of materials”, says Caro. Next, the team plan to extend their technique to include a broader range of materials and other types of spectroscopy.